Model-Informed Drug Development Training Course
About the Course
Model-Informed Drug Development (MIDD) is a quantitative and scientifically rational approach to optimize medical product development and regulatory decision-making. The U.S. Food and Drug Administration (FDA) and other regulatory authorities globally have continuously invested in the advancement of these approaches. The application MIDD spans the continuum from the conception of a drug candidate through post-drug approval monitoring. MIDD approaches go beyond PK/PD, and can be both disease-specific, or disease agnostic. The application of MIDD approaches also span the development of biological compounds, small molecules, and digital health technologies. It is crucial in first-in-human (FIH) dose selection from preclinical data, optimizing clinical trial design, simulation of different dosing regimens, formulations and route of administrations. It is also essential for tailoring drug treatments for specialized populations, such as patients with compromised organ functions, disease status and concomitant treatments or illnesses. It is used for pediatric dose extrapolation — utilizing data collected in the adult population to minimize or eliminate the need to conduct further clinical trials on pediatric patients. To access this course, please visit the FDA YouTube page, here.
In this MIDD Training Course, you will learn about:
The current landscape of MIDD across the drug development and regulatory science spectrum
Pharmacometrics
In other words, the definition of modeling and simulation in the context of MIDD. This course will teach you the principles of pharmacokinetics (PK), the types of pharmacodynamic (PD) endpoints and types of models linking exposure to response.
How MIDD affects regulatory decisions
Case studies in MIDD in different scenarios
Including pediatric drug, anti-viral, vaccine and biologics development.
Applications, opportunities, benefits and challenges in MIDD approach
Checking sensitivities of results, to prior assumptions and uncertainties when applying MIDD to decision-making in drug development
Physiological-based pharmacokinetics (PBPK) modeling in clinical development
Acceleration of Phase 2 using the MIDD approach, as well as Solubility Process Quotient (QSP) modeling to predict safety and efficacy of a drug candidate before FIH.
Future considerations in MIDD
including Digital Health Technology (DHT), Real World Data/Evidence (RWD/RWE), Artificial Intelligence (AI) and Machine Learning (ML).
Please note that new registration is closed. If you have any questions, please reach out to MIDDTraining@c-path.org.
C-Path MIDD Training Course Co-Chairs
Shu Chin Ma serves as C-Path Vice President, Model-Informed Drug Development and Quantitative Medicine. Shu Chin comes from a varied background of academia, regulatory, consultancy and the pharmaceutical industry. In her previous industry roles, she served as the head of clinical pharmacology and pharmacometrics, leading teams of scientists in therapeutic areas including rare, neurodegenerative and metabolic diseases, and blood and solid oncology from FIH dose selection to NDA filings.
Shu Chin was a part of the Certara Strategic Consultancy where she worked with various client companies on gap analysis, trial design, pharmacometrics analyses and regulatory filings. She was an ORISE fellow at Division of Pharmacometrics at the U.S. Food and Drug Administration and worked as a primary reviewer at the office of generic drugs prior to joining Certara. Shu Chin received a Master of Science degree from the Department of Chemical and Biomolecular Engineering at Cornell University, a Master of Philosophy from the department of Electrical Engineering at Yale University and a Ph.D. in Biomedical Engineering also from Yale. Dr. Ma is passionate about novel solutions to expedite safe drug development process.
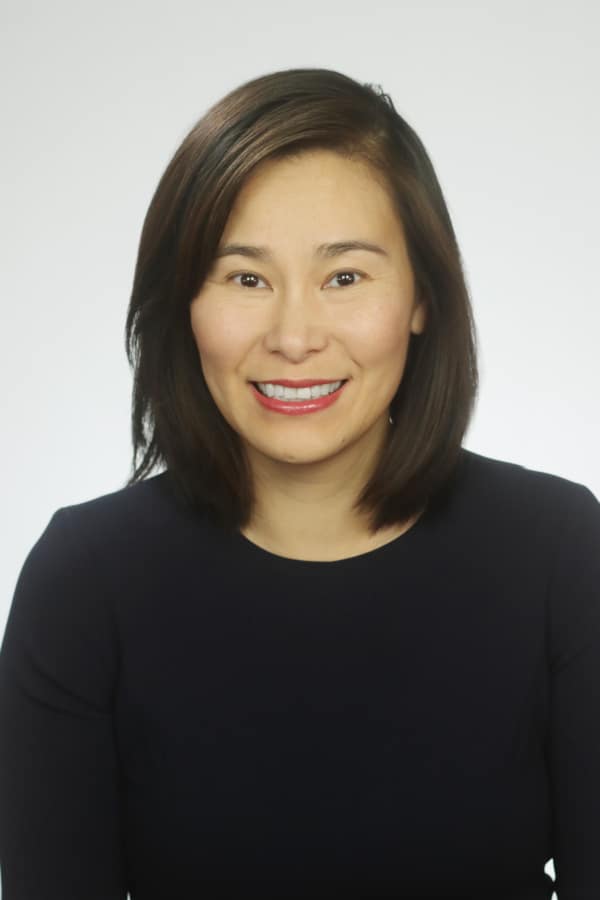
Shu Chin Ma, MSc, M.Phil, PhD, EMBA
Shu Chin Ma, MSc, M.Phil, PhD, EMBA
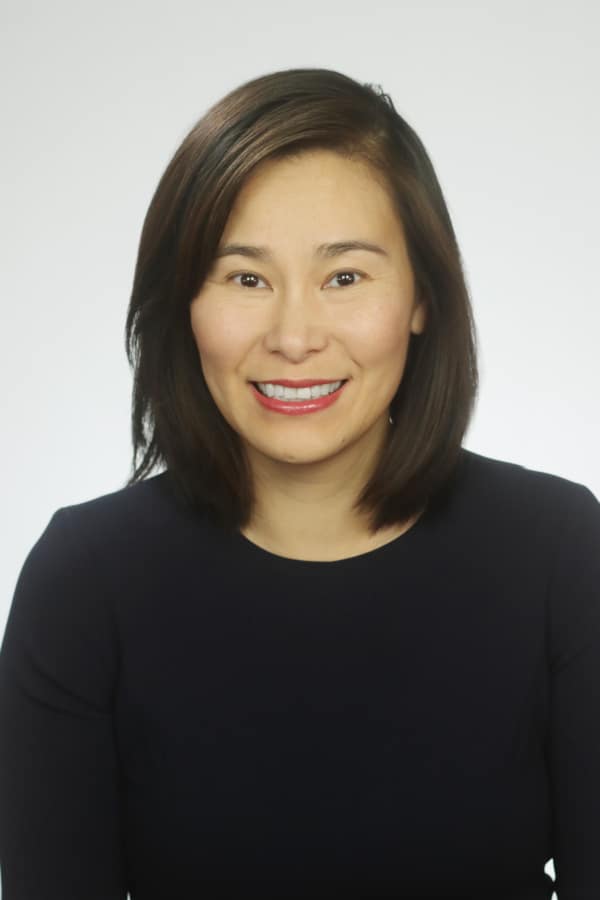
Shu Chin Ma, MSc, M.Phil, PhD, EMBA, serves as C-Path Vice President, Model-Informed Drug Development and Quantitative Medicine. Shu Chin comes from a varied background of academia, regulatory, consultancy, and the pharmaceutical industry. In her previous industry roles, she served as the head of clinical pharmacology and pharmacometrics, leading teams of scientists in therapeutic areas including rare, neurodegenerative and metabolic diseases, and blood and solid oncology from FIH dose selection to NDA filings. Shu Chin was a part of the Certara Strategic Consultancy where she worked with various client companies on gap analysis, trial design, pharmacometrics analyses, and regulatory filings. She was an ORISE fellow at Division of Pharmacometrics at the U.S. Food and Drug Administration and worked as a primary reviewer at the office of generic drugs prior to joining Certara. Shu Chin received a Master of Science degree from the Department of Chemical and Biomolecular Engineering at Cornell University, a Master of Philosophy from the department of Electrical Engineering at Yale University and a PhD in Biomedical Engineering also from Yale. Dr. Ma is passionate about novel solutions to expedite safe drug development process.
Training Module Titles and Instructors
Module Title | Instructor/Contributor | Lesson(s) in Module |
---|---|---|
Introduction to MIDD Training Course | Dr. Jeff Barrett (Aridhia) Dr. Qi Liu (FDA) | |
Overview — Model-Informed Drug Development | Dr. Jeff Barrett (Aridhia) | |
Module 1 – MIDD Landscape for Drug Development and Regulatory Sciences | Dr. Jeff Barrett (Aridhia) | |
Module 2 – Review of the Various Model Types Commonly Used for MIDD | Dr. Stacey Tannenbaum (Metrum Research Group) | Part 1 Part 2 Case studies |
Module 3 – How do models inform drug development and regulatory decisions | Dr. Colin Pillai (Pharmacometrics Africa/CP+ Associates) Dr. Jeff Barrett (Aridhia) | MIDD Impact and Decision-MakingMIDD Impact and Decision Making: Pediatric Drug Development and Considerations |
Module 4 – Foundation to Model-Informed Drug Development (MIDD) and Model Interconnectedness | Dr. Amy Cheung (Certara) Dr. Rajesh Krishna (Certara) Dr. Adekemi Taylor (Certara) | Foundation to Model-Informed Drug Development (MIDD) and Model Interconnectedness Case Study 1 Case Study 2 Case Study 3 Case Study 4 Case Study 5 Case Study 6 |
Module 5 – Model-Informed Decision Making in Drug Development: Part 1 | Dr. Marc Gastonguay (Metrum Research Group) | Why Use Models to Inform Decisions? Developing MIDD Strategies for Drug Development Decision Making |
Module 6 – Model-Informed Decision Making in Drug Development: Part 2 | Dr. Marc Gastonguay (Metrum Research Group) | Applying MIDD Strategies to Decision MakingChecking Sensitivity of Results to Assumptions and Uncertainties |
Module 7 – Examples and Vignettes | Dr. Dinko Rekic (AstraZeneca) Dr. Pascal Chanu (Genentech/Roche) Dr. Graham Lockwood (Sanofi) | Optimizing C.E.R.A. Pediatric Drug DevelopmentModel-Informed Clinical Development of MosunetuzumabAcceleration of Phase 2 Study through the MIDD Pilot ProgramMIDD to establish isatuximab (SARCLISA®) combination dosing regimen in relapsed/refractory multiple myeloma patientsApplication of PBPK Models in Eliglustat Clinical DevelopmentCaplacizumab® model-based dosing recommendations in pediatric patients with acquired thrombotic thrombocytopenic purpuraQSP modeling to predict safety and efficacy of a trispecific T cell engager antibody for multiple myeloma before FIH |
Module 8 – Future Consideration in MIDD including Digital Health, RWD/RWE and AI/ML | Dr. Jagdeep Podichetty (C-Path) Dr. Sakshi Sardar (C-Path) | Chapters 1 and 2: Digital Health Technologie Chapters 3 and 4: Artificial Intelligence and RWD/RWE |